Every year, the Foundation for Energy Informatics awards excellent bachelor's and master's theses that contribute to shaping the energy system of tomorrow. The foundation has awarded two theses completed in the Bits-to-Energy Lab in succession (both in 2021 and 2022) with a sponsorship prize in the category "Best master's thesis".
Elena Giacomazzi's master's thesis on "Forecast of electricity demand and load peaks in the distribution network with LSTM and transformer architectures" won a sponsorship award in 2022 (supervisors: Dr. Konstantin Hopf, Felix Haag).
Abstract:
Short-term load forecasting is an essential problem for the electric power industry. It contributes to operating power systems efficiently and making them more adaptable for using renewable energy. This thesis evaluates the performances of a long-short term memory (LSTM) neural network, a basic Transformer model, and the Temporal Fusion Transformer (TFT) on an hourly day-ahead and week-ahead forecasting task. Additionally, the models are evaluated for their accuracy in predicting load peaks. This thesis contributes to the literature by applying Transformer-based architectures to short-term load forecasting, which are relatively new to the field. Experiments are conducted on a data set of German household loads which is aggregated to the distribution network level. This work finds that the LSTM architecture performs best for the day-ahead horizon with MAPE = 6.09%, while the TFT performs best for the week-ahead forecast with MAPE = 6.46%. Although the results demonstrate the value of modern deep learning methods for load forecasting, further work is necessary to validate the methods on other data sets.
Felix Haag won a sponsorship award in 2021 for his master's thesis on "Explainable AI for in-depth benchmarking of domestic electricity consumption" (supervisors: Prof. Dr. Thorsten Staake, Dr. Konstantin Hopf).
Abstract:
Machine learning models have made strong advances in improving modeling capabilities. Such models can detect patterns in data and provide precise predictions, but are usually—compared to more traditional statistical methods such as linear regression—non-interpretable to humans. Motivated by this tension, research has put forth Explainable Artificial Intelligence (XAI) techniques that represent patterns discovered by machine learning in a human-readable way. One application area that can benefit from high predictive performance and the explanation of patterns in data is energy benchmarking. In the field, feedback of energy benchmarks has so far been limited to a single performance score. Thus, it remains unclear to feedback recipients which building and household characteristics affect their specific consumption. Taking a linear regression model as baseline, this thesis tests the applicability of XAI methods for energy benchmarking in the context of domestic electricity use in order to determine influencing factors. The results of this work show that XAI is able to identify building and household characteristics that are, according to a machine learning model, relevant for electricity consumption. Notably, XAI in combination with machine learning offers a higher predictive performance compared to a statistical approach while maintaining explainability. Energy benchmarking providers should therefore use XAI to offer additional information on performance ratings to improve the feedback given.
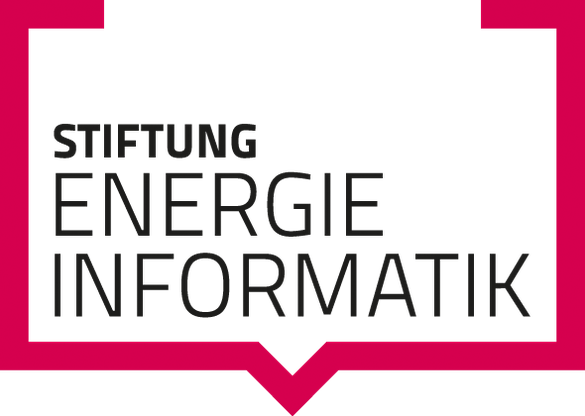
Write a comment